Fade2D is a powerful C++ Delaunay triangulation library known for its speed and robustness. In this benchmark, we explore its performance in triangulating varying point cloud sizes.
Performance and Settings
- Fade2D features a “Fast Mode” for accelerated triangulation of raster points, particularly beneficial for grid-aligned points, where computationally expensive predicates are much more common compared to other scenarios. This mode significantly enhances raster data triangulation speed.
- Fade2D offers multithreading support, enabling efficient utilization of multiple CPU cores. By default, Fade operates in single-threaded mode, which avoids nested multithreading in parallel applications. If you want multithreading, you can activate it explicitly using the free function setGlobalNumCPU(int numCPU)
- Performance scales almost linearly with point cloud size, with an upper bound of O(n*log(n)).
Benchmark Code
// * Init * std::vector<std::pair<std::string,int> > vNumPoints; vNumPoints.push_back(make_pair("numPoints: 1k",1000)); vNumPoints.push_back(make_pair("numPoints: 50k",50000)); vNumPoints.push_back(make_pair("numPoints: 100k",100000)); vNumPoints.push_back(make_pair("numPoints: 500k",500000)); vNumPoints.push_back(make_pair("numPoints: 1 mio",1000000)); vNumPoints.push_back(make_pair("numPoints: 10 mio",10000000)); //vNumPoints.push_back(make_pair("numPoints: 50 mio (10.7 GB)",50000000)); //vNumPoints.push_back(make_pair("numPoints: 100 mio (21.3 GB)",100000000)); //vNumPoints.push_back(make_pair("numPoints: 250 mio (53 GB)",250000000)); //vNumPoints.push_back(make_pair("numPoints: 500 mio (109 GB)",500000000)); // * Test * for(size_t i=0;i<vNumPoints.size();++i) { std::string label(vNumPoints[i].first); int numPoints(vNumPoints[i].second); // * Step 1 * Create a Fade object, make performance settings Fade_2D dt; dt.setFastMode(false); // Use 'true' for raster points (performance!) int numUsedCPU=setGlobalNumCPU(0); // 0 means: autodetect // * Step 2 * Prepare a vector of random points vector<Point2> vInPoints; generateRandomPoints(numPoints,0,100,vInPoints,1); // * Step 3 * Measure the triangulation time cout<<"\n"<<label<<": start"<<endl; double elapsed=dt.measureTriangulationTime(vInPoints); cout<<"Elapsed time (cores: "<<numUsedCPU<<"): "<<elapsed<<endl<<endl; }
- Step 1: Create an empty Fade_2D object and configure two important performance settings:
Fade_2D::setFastMode(bool b)
enables or disables a triangulation mode that significantly speeds up the triangulation of raster points.setGlobalNumCPU(int numCPU)
sets the number of CPU cores to be used. The special valuenumCPU=0
means autodetection and utilization of the available cores.
- Step 2: Prepare a vector of random points
- Step 3: Insert the points and measure the triangulation time.
Benchmarking Single- and Multithreaded Delaunay Triangulation
In this section, we take a closer look at benchmarking Fade2D’s performance, both in single-threaded and multithreaded scenarios. The benchmark results presented below showcase Fade2D’s remarkable speed and scalability.
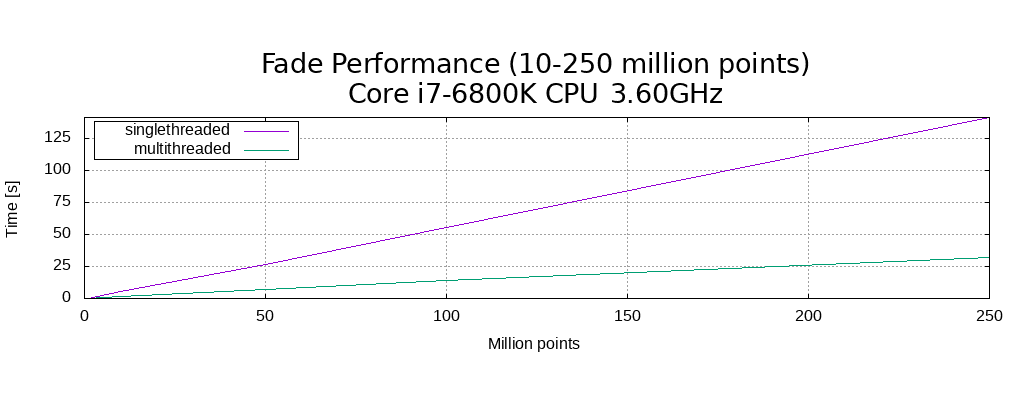
We tested Fade2D’s capabilities with varying point cloud sizes, ranging up to an impressive 250 million points. This translates to a staggering 500 million output triangles. The results show a remarkable almost linear relationship with the point cloud’s size, with an upper bound on the algorithm’s runtime being O(n*log(n)). On a 6-core CPU, multithreading delivers an impressive speedup of 4.39.
Raspberry PI – Still Impressive Performance
Fade2D isn’t just limited to high-end systems; it runs also on resource-efficient platforms like the Raspberry Pi which features a 32-bit ARM Cortex-A7 CPU and operates on a mere 3 watts of power, Fade2D demonstrated its capabilities by triangulating one million points (resulting in 2 million triangles) in just 7 seconds.

Getting accurate Benchmark Results
- Compile the benchmark as a x64 application in Release mode for better performance and because 32-bit applications suffer from the (MSVC 32-bit) 2GB memory limit.
- Run the test directly from the command prompt rather than within an IDE’s debug window.
- Ensure your system has sufficient RAM for the benchmark. On 64-bit systems, you’ll need 212 MB per million points, equivalent to 2 million triangles.
- Prevent cpu throttling by disabling energy-saving features during the test. Under Linux, use the Performance Governor and if you’re using a laptop, ensure it’s connected to a power source.
- Note that while Fade continues to support older environments like VS2010 and CentOS6.4, multithreading is disabled in those cases.
The next article Example2 – Traversing teaches you the important basics you need to access specific elements in a triangulation.
2 replies on “2D Delaunay Triangulation Benchmark – Example1”
Hey there!
Have you ever thought about parallelizing your algorithm on the gpu?
Making Fade2D even faster would be nice. But it would also add dependencies and thereby worsen the usability and compatiblity. Another thought is that Fade2D creates already ~15 mio. triangles per second (on recent hardware) and I think only very few projects have so much data that they require a new algorithm.